Semi-plenary Speakers
Katsuki Fujisawa
Mirjam Dür
Caroline Uhler
Yu-hong Dai
Erick Delage
Elad Hazan
Rachel Ward
Jonathan Kelner
Abstract and Short Biography
Katsuki Fujisawa
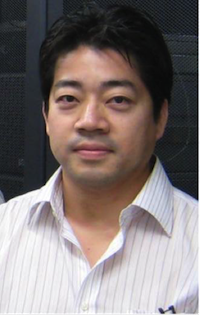
- Talk Title: High-performance and power-efficient computing for large-scale optimization problem and its new applications
- Abstract:
- Short Biography:
In this talk, we present our ongoing research project. The objective of this project is to develop advanced computing and optimization infrastructures for extremely large-scale graphs on post peta-scale supercomputers. We explain our challenge to Graph 500 and Green Graph 500 benchmarks that are designed to measure the performance of a computer system for applications that require irregular memory and network access patterns. In 2014 and 2015, our project team was a winner of the 8th,10th, and 11th Graph500 and the 3rd to 6th Green Graph500 benchmarks, respectively. We present our parallel implementation for large-scale SDP (SemiDefinite Programming) problem. The semidefinite programming (SDP) problem is a predominant problem in mathematical optimization. The primal-dual interior-point method (PDIPM) is one of the most powerful algorithms for solving SDP problems, and many research groups have employed it for developing software packages. We solved the largest SDP problem (which has over 2.33 million constraints), thereby creating a new world record. Our implementation also achieved 1.774 PFlops in double precision for large-scale Cholesky factorization using 2,720 CPUs and 4,080 GPUs on the TSUBAME 2.5 supercomputer. We have also started another research project for developing the Urban OS (Operating System). The Urban OS gathers big data sets of people and transportation movements by utilizing different sensor technologies and storing them to the cloud storage system. The Urban OS employs the graph analysis system developed by our research project above and provides a feedback to a predicting and controlling center to optimize many social systems and services. we briefly explain our ongoing research project for realizing the Urban OS.
Fujisawa has been a Full Professor at the Institute of Mathematics for Industry (IMI) of Kyushu University and a research director of the JST (Japan Science and Technology Agency) CREST (Core Research for Evolutional Science and Technology) post-Peta High Performance Computing. He received his Ph. D. from the Tokyo Institute of Technology in 1998. The objective of the JST CREST project is to develop an advanced computing and optimization infrastructure for extremely large-scale graphs on post peta-scale super-computers. His project team has challenged the Graph500 and Green Graph500 benchmarks, which are designed to measure the performance of a computer system for applications that require irregular memory and network access patterns. In 2014 and 2015, his project team was a winner of the 8th,10th, and 11th Graph500 and the 3rd to 6th Green Graph500 benchmarks, respectively.
Mirjam Dür
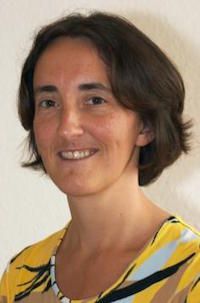
- Talk Title: Copositive Optimization
- Abstract:
- Short Biography:
A copositive optimization problem is a problem in matrix variables with a constraint which requires that the matrix be in the copositive cone. This means that its quadratic form takes nonnegative values over the nonnegative orthant. By definition, every positive semidefinite matrix is copositive, and so is every entrywise nonnegative matrix, but the copositive cone is significantly larger than both the semidefinite and the nonnegative matrix cones.
Many combinatorial problems like for example the maximum clique problem can be equivalently formulated as a copositive problem. Burer (2009) showed that also any nonconvex quadratic problem with linear constraints and binary variables can be reformulated as such a copositive problem. This is remarkable, since by this approach a nonconvex problem is reformulated equivalently as a convex problem. The complexity of the original problem is entirely shifted into the cone constraint.
We review recent progress in this copositive approach, concerning both theoretical results and numerical issues.
Mirjam Dür was born in Vienna, Austria, where she received a M.Sc. degree in Mathematics from the University of Vienna in 1996. She received a PhD in applied mathematics from University of Trier (Germany) in 1999. After that, she worked as an assistant professor at Vienna University of Economics and Business Administration, as a junior professor at TU Darmstadt (Germany), and as a Universitair Docent at the University of Groningen (The Netherlands). Since October 2011, she is a professor of Nonlinear Optimization at the University of Trier, Germany.
Prof. Dür is a member of the editorial boards of the journals Mathematical Methods of Operations Research, Journal of Global Optimization, and Optimization Methods and Software, and of the book series Springer Briefs in Optimization. In 2010, she was awarded a VICI-grant by the Dutch Organisation for Scientific Research (NWO), and in 2012 a GIF Research grant by the German-Israeli Foundation for Scientific Research and Development. As of 2016, she is one of the principal investigators in the Research Training Group Algorithmic Optimization at the University of Trier.
Caroline Uhler
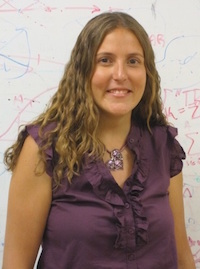
- Talk Title: Totally positive exponential families and graphical models
- Abstract:
- Short Biography:
We study maximum likelihood estimation for exponential families that are multivariate totally positive of order two (MTP2). Such distributions appear in the context of ferromagnetism in the Ising model and various latent models, as for example Brownian motion tree models used in phylogenetics. We show that maximum likelihood estimation for MTP2 exponential families is a convex optimization problem. For quadratic exponential families, such as Ising models and Gaussian graphical models, we show that MTP2 implies sparsity of the underlying graph without the need of a tuning parameter. Moreover, we show that the maximum likelihood estimator always exists even in the high-dimensional setting. These properties make MTP2 constraints an intriguing alternative to methods for learning sparse graphical models such as the graphical lasso.
# The title and abstract of the semi-plenary talk by Caroline Uhler have been changed and are different from those in the conference booklet.
Caroline Uhler is an assistant professor in EECS and IDSS at MIT. She holds an MSc in Mathematics, a BSc in Biology, and an MEd in High School Mathematics Education from the University of Zurich. She obtained her PhD in Statistics from UC Berkeley in 2011. After short postdoctoral positions at the Institute for Mathematics and its Applications at the University of Minnesota and at ETH Zurich, she joined IST Austria as an assistant professor (2012-2015). Her research focuses on mathematical statistics, in particular on graphical models and the use of optimization, algebraic and geometric methods in statistics, and on applications to biology. She is an elected member of the International Statistical Institute and she received a Sofja Kovalevskaja Award from the Humboldt Foundation and a START Award from the Austrian Science Fund.
Yu-hong Dai
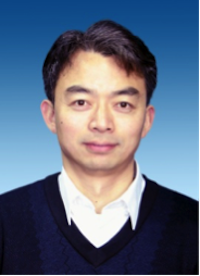
- Talk Title: The Steepest Descent and Conjugate Gradient Methods Revisited.
- Abstract:
- Short Biography:
The steepest descent and conjugate gradient methods are basic first order methods for unconstrained optimization. More efficient variants have been proposed in recent decades by forcing them to approximate Newton's method (or quasi-Newton method). In this talk, I shall review some recent advances on steepest descent method and conjugate gradient method. While significant numerical improvements have been made, the behavior of these more efficient variants are still to be understood and more analysis are obviously required.
Yu-Hong Dai received his bachelor degree in applied mathematics from the Beijing Institute of Technology, China, in 1992. Then he studied nonlinear optimization in the Institute of Computational Mathematics, Chinese Academy of Sciences (CAS), and received his doctor degree in 1997. Now he is Feng Kang distinguished professor of Academy of Mathematics and Systems Science (AMSS) of CAS. Currently, he is also assistant president of AMSS and Vice-Director of Center for Optimization and Applications (COA) of AMSS.
His research interests mainly lie in nonlinear optimization and various optimization applications. Specifically, he is quite interested in proposing simple but efficient optimization methods (for example, the Dai-Yuan conjugate gradient method) and in providing theoretical properties for existing elegant optimization methods (for example, the perfect example for the failure of the BFGS method and the R-linear convergence of the Barzilai-Borwein gradient method). He has published many papers in various journals, including Mathematical Programming, SIOPT, SIIS, SIMAX, ITSP, Mathematics of Computation, Numerische Mathematics, IMA Journal on Numerical Analysis and Journal of the OR Society of China. Because of his accomplishments, he received the Fifth ZhongJiaQing Mathematics Award (1998), Second Prize of the National Natural Science of China in 2006 (Rank 2), the Tenth Science and Technology Award for Chinese Youth (2007), Best Paper Award of International Conference on Communication (2011), the China National Funds for Distinguished Young Scientists (2011), Feng Kang Prize of Scientific Computing (2015). Dr. Dai is currently the president of Chinese Mathematical Programming Subsociety of ORSC. Meanwhile, he has held editorial positions for several journals, including International Transactions in Operational Research (ITOR), Asia Pacific Journal of Optimization (APJOR), Science China: Mathematics (SciChina:Math), Journal of the OR Society of China.
Erick Delage
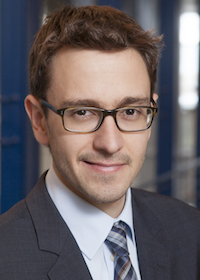
- Talk Title: Preference robust optimization for decision making under uncertainty
- Abstract:
- Short Biography:
Decisions often need to be made in situations where parameters of the problem that is addressed are considered uncertain. While there are a number of well-established paradigms that can be used to design an optimization model that accounts for risk aversion in such a context (e.g. using expected utility or convex risk measures), such paradigms can often be impracticable since they require a detailed characterization of the decision maker’s perception of risk. Indeed, it is often the case that the available information about the DM’s preferences is both incomplete, because preference elicitation is time consuming, and imprecise, because subjective evaluations are prone to a number of well-known cognitive biases. In this talk, we introduce preference robust optimization as a way of accounting for ambiguity about the DM’s preferences. In a financial environment, an optimal preference robust investment will have the guarantee of being preferred to the largest risk-free return that could be made available. We show how preference robust optimization models are quasiconvex optimization problems of reasonable dimension when parametric uncertainty is described using scenarios and preference information takes the form of pairwise comparisons of discrete lotteries. Finally, we illustrate numerically our findings with a portfolio allocation problem and discuss possible extensions.
Erick Delage completed his Ph.D. at Stanford University in 2009, is currently associate professor in the Department of Decision Sciences at HEC Montreal, and was recently appointed as chairholder of Canada Research Chair in Decision Making Under Uncertainty. He serves on the editorial board of Management Science, Pacific Journal of Optimization, and Computational Management Science where he recently co-edited a special issue on recent advances in the field of robust optimization. His research interests span the areas of robust optimization, stochastic programming, decision theory, artificial intelligence and applied statistics.
Elad Hazan
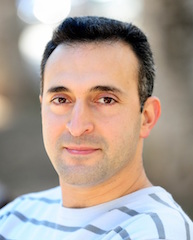
- Talk Title: Simulated Annealing with an Efficient Universal Barrier
- Abstract:
- Short Biography:
Interior point methods and random walk approaches have been long considered disparate approaches for convex optimization. We show how simulated annealing, one of the most common random walk algorithms, is equivalent, in a certain sense, to the central path interior point algorithm applied to the enhanced entropic universal barrier function. Using this observation we improve the state of the art in polynomial time convex optimization in the membership-oracle model.
Elad Hazan is a professor of computer science at Princeton University. Previously he had been an associate professor of operations research at the Technion. His research focuses on the design and analysis of algorithms for basic problems in machine learning and optimization. Amongst his contributions are the co-development of the AdaGrad algorithm for training learning machines, and the first sublinear-time algorithms for convex optimization. He is the recipient of (twice) the IBM Goldberg best paper award in 2012 for contributions to sublinear time algorithms for machine learning, and in 2008 for decision making under uncertainty, a European Research Council grant , a Marie Curie fellowship and a Google Research Award (twice). He serves on the steering committee of the Association for Computational Learning and has been program chair for COLT 2015.
Rachel Ward
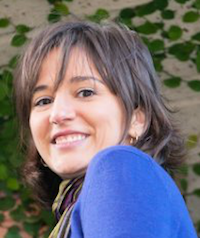
- Talk Title: Clustering subgaussian mixtures by semidefinite programming
- Abstract:
- Short Biography:
We introduce a model-free relax-and-round algorithm for k-means clustering based on a semidefinite relaxation of the (NP-hard) k-means optimization problem. The algorithm interprets the SDP output as a denoised version of the original data and then rounds this output to a hard clustering. We provide a generic method for proving performance guarantees for this algorithm, and we analyze the algorithm in the context of subgaussian mixture models. We also study the fundamental limits of estimating Gaussian centers by k-means clustering in order to compare our approximation guarantee to the theoretically optimal k-means clustering solution. This is joint work with Dustin Mixon and Soledad Villar.
Rachel Ward received the B.Sc. degree in mathematics from the University of Texas at Austin in 2005 and the Ph.D. degree in applied and computational mathematics in 2009 from Princeton University. After working as a Post-Doctoral Fellow at the Courant Institute, New York University, she joined the University of Texas at Austin in 2011 as an Assistant Professor of mathematics. She received the Alfred P Sloan Research Fellowship, the Donald D. Harrington Faculty Fellowship, and the NSF CAREER Grant. Her research interests include image processing, statistical machine learning, optimization, compressed sensing, and quantization.
Jonathan Kelner
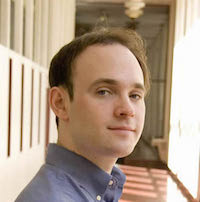
- Talk Title: Bridging the Continuous and the Combinatorial: Emerging Tools, Techniques, and Design Principles for Graph Algorithms and Convex Optimization
- Abstract:
- Short Biography:
Flow and cut problems on graphs are among the most fundamental and extensively studied problems in Operations Research and Optimization, playing a foundational role in both the theory and practice of algorithm design. While the classical algorithms for these problems were largely based on combinatorial approaches, the past several years have witnessed the emergence of a powerful collection of new approaches rooted in continuous optimization. These have allowed researchers to provide better provable algorithms for a wide range of graph problems, in some cases breaking algorithmic barriers that had stood for several decades.
The key to these improvements has been the development of a set of techniques for systematically linking the combinatorial structure of a graph problem to the numerical and geometric properties of the corresponding convex program, and for exploiting this connection to design iterative methods with improved guarantees. This relationship between graph theory and continuous optimization has proven fruitful in the other direction as well, leading to fast algorithms and new insights for solving a variety of problems in convex optimization, computational linear algebra, and the analysis of random processes.
In this talk, I will survey some of the main results, recurring themes, and technical tools that arise in this confluence of fields, and I will illustrate these by sketching how they can be used to find approximately maximum flows on undirected graphs in close to linear time. I will then discuss some recent results and highlight several challenges and open problems that I believe are within reach.
Jonathan Kelner is an Associate Professor of Applied Mathematics in the MIT Department of Mathematics and a member of the MIT Computer Science and Artificial Intelligence Laboratory (CSAIL). His research focuses on the application of techniques from pure mathematics to the solution of fundamental problems in algorithms and complexity theory. He was an undergraduate at Harvard University, and he received his Ph.D. in Computer Science from MIT in 2006. Before joining the MIT faculty, he spent a year as a member of the Institute for Advanced Study. He has received a variety of awards for his work, including an NSF CAREER Award, an Alfred P. Sloan Research Fellowship, the Best Paper Awards at STOC 2011, SIGMETRICS 2011, and SODA 2014, the Harold E. Edgerton Faculty Achievement Award, and the MIT School of Science Teaching Prize.