Plenary Speakers
Florian Jarre
Shuzhong Zhang
Jong-Shi Pang
Francis Bach
Abstract and Short Biography
Florian Jarre
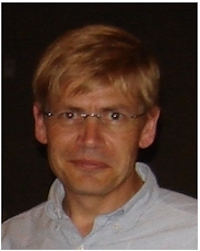
- Talk Title: Nonlinear Minimization Techniques Without Using Derivatives
- Abstract:
- Short Biography:
It is mostly a matter of laziness -- but with imporant implications:
We discuss possible applications of minimization without (explicitly) using derivatives. While automatic differentiation offers an elegant and efficient alternative in many cases, due to its simplicity, the minimization without using derivative information is interesting in several respects:
On the one side the applications mentioned above, on the other side a slight change in the use of the tools for minimization.
More precisely, we consider the problem of finding a local optimal solution to an optimization problem with a smooth objective function and smooth constraints, but without the availability of derivative informations. There is a wealth of methods tuned to very expensive and/or noisy function evaluations, and there are methods in Matlab/Octave such as fmincon, fminunc, or minFunc that are tailored to situations where the derivative information is provided, and that use finite differences when derivatives are unavailable.
We discuss modifications of the latter approach taking into account the fact that finite differences are numerically expensive compared to standard matrix operations. In particular, we consider a new line search based on least squares spline functions, a new finite difference setup, and a Sl_2QP method for constrained minimization. Some numerical examples conclude the talk.
Florian Jarre is Professor of Mathematics at the Heinrich-Heine-Universitaet Duesseldorf, Germany, and leads the Optimization Group in Duesseldorf. He received his PhD from the University of Wuerzburg, Germany in 1989. Dr. Jarre's research is concerned with interior point methods for convex optimization, large scale approaches for solving convex conic programs, and applications of semidefinite and completely positive programming. His recent interest are applications of optimization without using derivatives. He is an Associate Editor of Optimization Methods and Software and Optimization and Engineering.
Shuzhong Zhang
- Talk Title: Variants of the ADMM and Their Convergence Properties
- Abstract:
- Short Biography:
The alternating direction method of multipliers (ADMM) proved to be a remarkably stable and effective solution approach to solve many structured convex optimization models. In the past few years, an intensive stream of research effort has been paid to the performance of the ADMM and its many variants designed to accommodate various formulations arising from applications. In this talk, we shall survey several aspects of the afore-mentioned research effort, and present some new results including the convergence of a randomized multi-block ADMM.
Shuzhong Zhang is Professor and Head of Department of Industrial and System Engineering, University of Minnesota. He received a B.Sc. degree in Applied Mathematics from Fudan University in 1984, and a Ph.D degree in Operations Research and Econometrics from the Tinbergen Institute, Erasmus University, in 1991. He had held faculty positions at Department of Econometrics, University of Groningen (1991-1993), and Econometric Institute, Erasmus University (1993-1999), and Department of Systems Engineering & Engineering Management, The Chinese University of Hong Kong (1999-2010). He received the Erasmus University Research Prize in 1999, the CUHK Vice-Chancellor Exemplary Teaching Award in 2001, the SIAM Outstanding Paper Prize in 2003, the IEEE Signal Processing Society Best Paper Award in 2010, and the 2015 SPS Signal Processing Magazine Best Paper Award. Dr. Zhang was an elected Council Member at Large of the MPS (Mathematical Programming Society) (2006-2009), and served as Vice-President of the Operations Research Society of China (ORSC) (2008-2012). He serves on the Editorial Board of several academic journals, including Operations Research, and Management Science.
Jong-Shi Pang
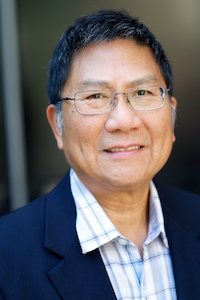
- Talk Title: Difference-of-Convex Optimization for Statistic Learning
- Abstract:
- Short Biography:
We address a fundamental bi-criteria optimization problem for variable selection in statistical learning; the two criteria being a loss func- tion which measures the fitness of the model and a penalty function which controls the complexity of the model. Motivated by the increased interest in non-convex surrogates of the step l0-function that counts the number of nonzero components of the model variables, we show that many well-known sparsity functions existed in the literature admit a unified difference-of- convex(dc) representation that facilitates systematic analysis and algorith- mic development. Such a representation involves non-differentiable functions and thus understanding the associated optimization problems require care. Two classes of sparsity functions are considered: exact versus surrogate. Exact sparsity functions are those whose zeros coincide with the sparsity pattern of the model unknowns; surrogate sparsity functions are those that are substitutes for the l0-function. We derive several theoretical results on the non-convex Lagrangian formulation of the bi-criteria optimization, re- lating it with a penalty constrained formulation in terms of their prospective computable stationary solutions, and giving conditions under which a direc- tional stationary solution of the Lagrangean problem is a global minimizer. We present computational algorithms for solving these bi-criteria dc opti- mization problems and present numerical results using data sets existed in the literature. The results demonstrate the superiority of using a non-convex formulation over a convex approach on a variety of compressed sensing and binary classification problems.
Jong-Shi Pang joined the University of Southern California as the Epstein Family Professor of Industrial and Systems Engineering in August 2013. Prior to this position, he was the Caterpillar Professor and Head of the Department of Industrial and Enterprise Systems Engineering for six years between 2007 and 2013. He held the position of the Margaret A. Darrin Distinguished Professor in Applied Mathematics in the Department of Mathematical Sciences and was a Professor of Decision Sciences and Engineering Systems at Rensselaer Polytechnic Institute from 2003 to 2007. He was a Professor in the Department of Mathematical Sciences at the Johns Hopkins University from 1987 to 2003, an Associate Professor and then Professor in the School of Management from 1982 to 1987 at the University of Texas at Dallas, and an Assistant and then an Associate Professor in the Graduate School of Industrial Administration at Carnegie-Mellon University from 1977 to 1982. During 1999 and 2001 (full time) and 2002 (part-time), he was a Program Director in the Division of Mathematical Sciences at the National Science Foundation. Professor Pang was a winner of the 2003 George B. Dantzig Prize awarded jointly by the Mathematical Programming Society and the Society for Industrial and Applied Mathematics for his work on finite-dimensional variational inequalities, and a co-winner of the 1994 Frederick W. Lanchester Prize awarded by the Institute for Operations Research and Management Science. Several of his publications have received best paper awards in different engineering fields: signal processing, energy and natural resources, computational management science, and robotics and automation. He is an ISI Highly Cited Researcher in the Mathematics Category between 1980--1999; he has published 3 widely cited monographs and more than 100 scholarly journals in top peer reviewed journals. Dr. Pang is a member in the inaugural 2009 class of Fellows of the Society for Industrial and Applied Mathematics. Professor Pang's general research interest is in the mathematical modeling and analysis of a wide range of complex engineering and economics systems with focus in operations research, (single and multi-agent) optimization, equilibrium programming, and constrained dynamical systems.
Francis Bach
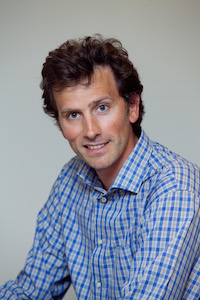
- Talk Title: Linearly-convergent stochastic gradient algorithms.
- Abstract:
- Short Biography:
Many machine learning and signal processing problems are traditionally cast as convex optimization problems where the objective function is a sum of many simple terms. In this situation, batch algorithms compute gradients of the objective function by summing all individual gradients at every iteration and exhibit a linear convergence rate for strongly-convex problems. Stochastic methods rather select a single function at random at every iteration, classically leading to cheaper iterations but with a convergence rate which decays only as the inverse of the number of iterations. In this talk, I will present the stochastic averaged gradient (SAG) algorithm which is dedicated to minimizing finite sums of smooth functions; it has a linear convergence rate for strongly-convex problems, but with an iteration cost similar to stochastic gradient descent, thus leading to faster convergence for machine learning problems. I will also mention several extensions, in particular to saddle-point problems, showing that this new class of incremental algorithms applies more generally.
Francis Bach is a researcher at Inria, leading since 2011 the Sierra project-team, which is part of the Computer Science Department at Ecole Normale Superieure. He completed his Ph.D. in Computer Science at U.C. Berkeley, working with Professor Michael Jordan, and spent two years in the Mathematical Morphology group at Ecole des Mines de Paris, then he joined the Willow project-team at INRIA/Ecole Normale Superieure from 2007 to 2010. Francis Bach is interested in statistical machine learning, and especially in graphical models, sparse methods, kernel-based learning, convex optimization, vision and signal processing. He obtained in 2009 a Starting Grant from the European Research Council and received in 2012 the INRIA young researcher prize. In 2015, he was program co-chair of the International Conference in Machine Learning (ICML).